
Communications and Signal Processing Seminar
Shannon's Information Measures and Markov Structures
Add to Google Calendar
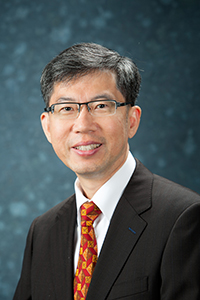
Originally studied in statistical physics, Markov random fields find applications in statistics, image processing, and in recent years social networks and big data. Most studies of finite Markov random fields assume that the underlying probability mass function (pmf) of the random variables is strictly positive. With this assumption, the probability mass function takes the form of a Gibbs measure which possesses many nice properties.
In general, non-strictly positive pmf's have very complicated conditional independence structure and are difficult to handle. An alleviation of this difficulty is to use conditional mutual information to characterize conditional mutual independencies. Specifically, for random variables X, Y, and Z, X and Y are independent conditioning on Z if and only if I(X;Y|Z)=0, regardless of whether the underlying pmf is strictly positive or not.
In the 1990's, the theory of I-Measure was developed as a full-fledged set-theoretic interpretation of Shannon's information measures. In this talk, we first give an overview of this theory. Then we discuss a set of tools developed on the I-Measure that is most suitable for studying a special Markov structure called full conditional mutual independence (FCMI), which turns out to be a building block for Markov random fields. One application of these tools is to show that the I-Measure of a Markov chain (a special case of a Markov random field) exhibits a very simple structure and is always nonnegative.
In the last part of the talk, we discuss some recent results along this line: i. a characterization of the Markov structure of a subfield of a Markov random field; ii. the Markov chain being the only Markov random field such that the I-Measure is always nonnegative.
Raymond W. Yeung received his PhD in electrical engineering from Cornell University. He was with AT&T Bell Laboratories from 1988 to 1991. Since 1991, he has been with The Chinese University of Hong Kong, where he is now Choh-Ming Li Professor of Information Engineering and Co-Director of Institute of Network Coding. His research interests include information theory and network coding. He is the author of the textbooks A First Course in Information Theory (Kluwer Academic/Plenum 2002) and its revision Information Theory and Network Coding (Springer 2008).
He was a recipient of the Croucher Foundation Senior Research Fellowship for 2000/2001, the Best Paper Award (Communication Theory) of the 2004 International Conference on Communications, Circuits and System, the 2005 IEEE Information Theory Society Paper Award, the Friedrich Wilhelm Bessel Research Award of the Alexander von Humboldt Foundation in 2007, and the 2016 IEEE Eric E. Sumner Award.