Herbert Winful part of MURI team investigating AI-guided self-organization in nonlinear systems
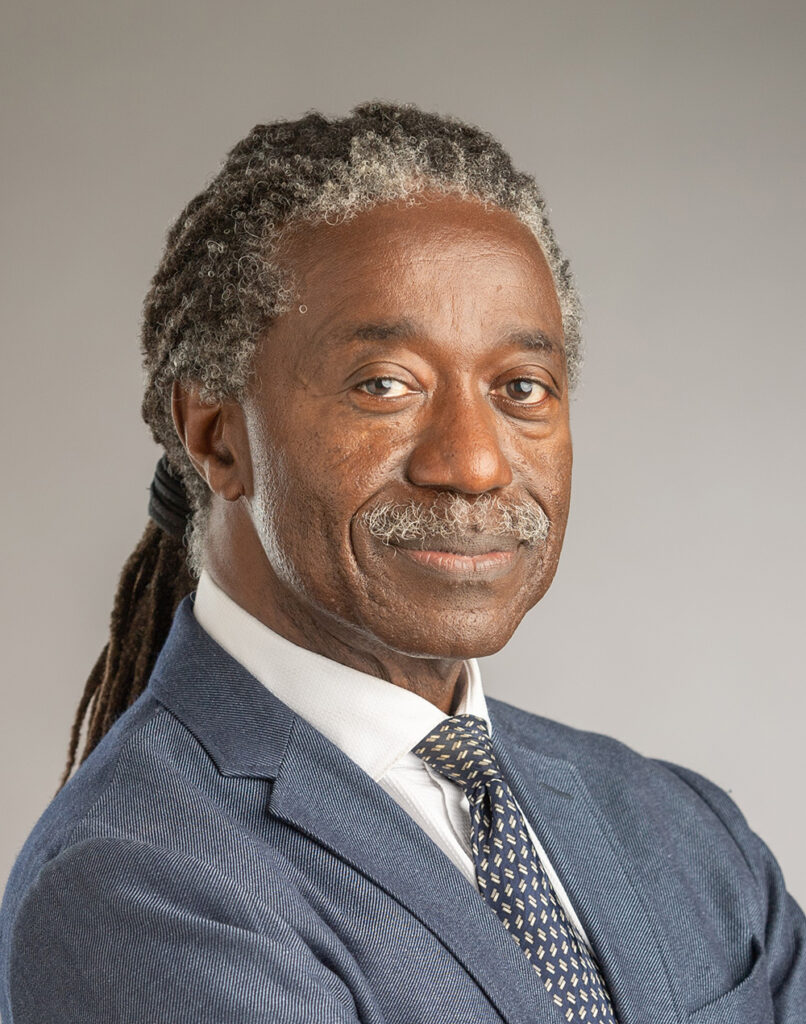
How can a group of fireflies synchronize their flashing to act as a single strobe light? How do heart pacemaker cells synchronize their firing to generate a regular heartbeat like clockwork? And how can a researcher get a set of semiconductor lasers to synchronize their emissions and act as one giant, high-powered laser? This last question is one that Professor Herbert Winful grappled with when he first joined the EECS faculty in January 1987—and that has become relevant again as he serves as co-PI on a new $9M, five year Multidisciplinary University Research Initiative (MURI) in collaboration with researchers at five other universities. The project, entitled “AI-Guided Self-Organization: Tailoring Disorder to Shape Complex Nonlinear Dynamics,” is led by Professors Hui Cao and Logan Wright at Yale University.
Self-organization through synchronization is a principle underlying the behaviour of complex systems, such as fireflies, neurons, lasers, or weather systems. Through local interactions and feedback, these nonlinear systems can organize themselves into regular spatial, temporal, or spatio-temporal structures that could not be predicted from the behavior of the individual elements.
“When such synchronization breaks down, the behavior is marvelously complex, often leading to chaotic dynamics—as seen, for example, in heart arrhythmia,” Winful said. “In this grant, we are using some new ideas from AI to control the self-organization in several electronic devices.”
Winful will bring his expertise in coupled laser arrays to the MURI, having spent his early career developing the theory still universally used to describe their behavior. He is currently working with an undergraduate, Zhanning (Justin) Liu, who, Winful noted, “is excellent and already functioning like an advanced graduate student on the project.” A newly-hired postdoc, Max Chumley, will also be joining the group in May.
The MURI team will use semiconductor laser arrays, degenerate cavity lasers, and multimode fiber lasers to model complex nonlinear dynamics. Other members of the team will also work with superconducting quantum interference devices (SQUIDs) and analog electronic oscillators. Using theory, digital twin simulations, and high-throughput experiments, they aim to develop and train a generalized artificial intelligence (AI) system to control and encourage cooperation between complex physical systems.
Enhancing cooperation between lasers could increase their combined output by the number of coupled lasers squared, with implications for new areas of plasma research—important for scaling nuclear fusion as a clean energy source—the development of next-generation x-rays, and more.
Their vision for this AI system is inspired by the Google DeepMind AI that learned to outperform the average human at Atari video games in 2015, and physics theory that they refer to as “smart disorder,” which suggests that “if disorder can be added to a physical system with sufficient care, structure, and scale, it may be possible to finally conquer the self-organization of real, messy physical systems.” Ultimately, they hope to develop something akin to a “large physics model” that, like ChatGPT predicts language, could predict the evolution of complex physical systems.
For Winful, this project also revives a hope that he kindled as a new faculty member joining ECE in January 1987—to synchronise the activity of an array of lasers, such that their output will compound into a single, superpowered burst. Even then, researchers had already been working on mastering this phenomenon, known as in-phase phase-locking, for decades without success. Winful was so singularly intrigued by this topic at the time, he selected his University of Michigan username to reflect it: arrays@umich, for the laser arrays he wanted to phase-lock.
“I thought it would be a temporary uniqname,” he recalled with a good-natured laugh, “but it has stuck with me for 38 years.”
Winful quickly hired a graduate student, S.S. Wang, to tackle the theoretical aspect of this challenge with him. Together, they derived and solved the equations that described the behavior of coupled semiconductor lasers. The result: random-looking but predictable behavior, known as chaos. Unlike noise, which is random and difficult to predict, chaos is governed by deterministic differential equations that describe how the system will evolve over time. Winful’s research also demonstrated spontaneous synchrony between subsets of chaotically-behaving lasers in an array, even when they were not phase-locked.
“I was used to studying nonlinear systems that can behave in strange ways,” he said. “My previous experience with nonlinear systems—single lasers—was that if you coupled them together, you could see instabilities that were not present in the uncoupled lasers. I approached the problem trying to see what interesting phenomena would occur and also try to understand why people had such a hard time getting laser arrays to operate in phase. I wanted to be able to crack that problem.”
After a couple of years, lacking a promising solution to the problem of stable in-phase phase-locking, Winful moved on to other problems. But this initial work, from 1987–1990, remains foundational to the field of laser array dynamics and led Cao and Wright to reach out to him in 2023 with a request to join their MURI team. Using AI to control the synchronization of the lasers and “shake” the system with targeted disorder into phase-locking could be the solution to Winful’s original problem.
“Once we achieve that, I can retire,” he joked.
In addition to Winful, Cao, and Wright, the MURI team includes Steven Anlage (University of Maryland College Park), Ying-Cheng Lai (Arizona State University), Vassilios Kovanis (Virginia Tech), and Tsampikos Kottos (Wesleyan University) as co-PIs. These collaborators have existing experience with experiments using machine learning to control complex physical systems in the laboratory.