
Communications and Signal Processing Seminar
Stochastic Majorize-Minimize Subspace Algorithm for Large Scale Data Processing
Add to Google Calendar
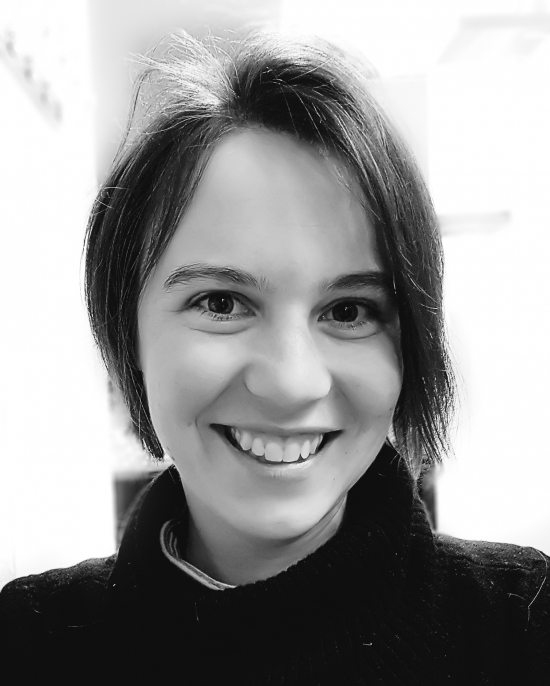
Stochastic approximation techniques play a prominent role in solving many large scale problems encountered in machine learning or image/signal processing. In these contexts, the statistics of the data are often unknown a priori or their direct computation is too intensive, and they have thus to be estimated online from the observations. For batch optimization of an objective function being the sum of a data fidelity term and a penalization (e.g. a sparsity promoting function), Majorize-Minimize (MM) methods have recently attracted much interest since they are fast, highly flexible, and effective in ensuring convergence. The goal of this talk is to show how these methods can be successfully extended to the case when the data fidelity term corresponds to a least squares criterion and the cost function is replaced by a sequence of stochastic approximations of it. In this context, we propose an online version of an MM subspace algorithm and we establish its convergence by using suitable probabilistic tools. We also provide new results on the convergence rate of such kind of algorithm. Numerical results illustrate the good practical performance of the proposed algorithm associated with a memory gradient subspace, when applied to both non-adaptive and adaptive linear system identification scenarios.
Emilie Chouzenoux is an assistant professor at the University of Paris-East Marne and head of the teaching department Métiers du Multimédia et de l'internet. She earned the Master Degree in Automatic and Production Systems from Ecole Centrale Nantes (with honors) and the Engineer Degree in Automatic from Ecole Centrale de Nantes in 2007. Her research focuses on algorithmic strategies for stepsize determination in iterative descent optimization methods in image and signal processing.