
Dissertation Defense
Solving Poisson Inverse Problems in Phase Retrieval and Single Photon Emission Computerized Tomography
This event is free and open to the publicAdd to Google Calendar
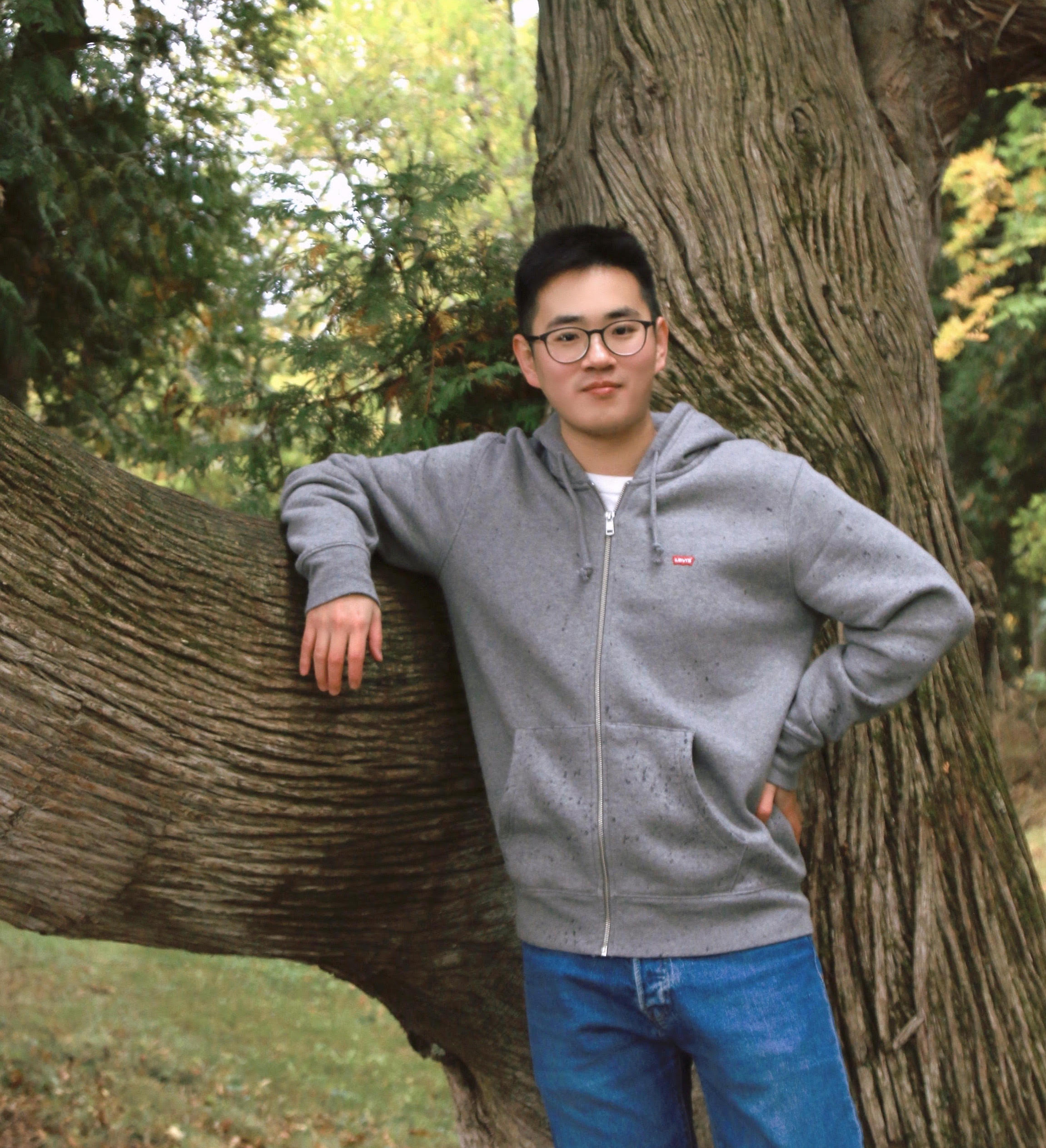
PASSCODE: zongyu
We live in a world where many objects cannot be imaged directly and hence rely on reconstruction algorithms to solve the corresponding inverse imaging problems. As the recorded photon arrivals by the sensor are often assumed to follow Poisson distributions, algorithms for solving Poisson inverse problems are crucial. This thesis tackles two applications where Poisson inverse problems arise: phase retrieval and single photon emission computerized tomography (SPECT). For phase retrieval, we propose novel optimization algorithms working in low-count regimes, including a novel majorize-minimize algorithm, a modified Wirtinger flow algorithm using the observed Fisher information for step size and a generative image prior based on score matching. Our proposed algorithms lead to faster convergence rate and improved reconstruction quality. For SPECT imaging, we focus on deep learning (DL) solutions including:
1) End-to-end training of unrolled iterative neural networks using our developed memory efficient Julia toolbox for SPECT image reconstruction.
2) DL algorithm for joint dosimetry estimation and image deblurring for estimating patient’s absorbed dose-rate distribution in radionuclide therapy.
3) Unsupervised coordinate-based learning for predicting missing SPECT projection views.
CHAIR: Jeffrey A. Fessler