
Communications and Signal Processing Seminar
Solving Corrupted Systems of Quadratic Equations, Provably
Add to Google Calendar
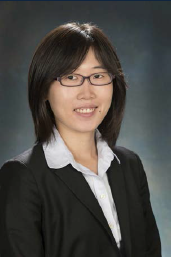
In this talk, we consider the problem of estimating a low-dimensional subspace by observing the magnitudes of the backprojections of a set of random vectors, which are quadratic in the unknown subspace. This problem is motivated by applications in covariance sketching of high-dimensional data streams, phase retrieval, quantum space tomography, to name a few. We will describe provable algorithms for solving this problem using both convex and non-convex approaches, even when the measurements are corrupted by arbitrary outliers. The class of convex approaches are based on lifting, and we will highlight a method for resisting outliers without regularization. The class of non-convex approaches are based gradient descent and its stochastic variant (namely, Kaczmarz method), and we will highlight a method for resisting outliers based on median-guided truncation.
Yuejie Chi is an assistant professor in the Department of Electrical and Computer Engineering and the Department of Biomedical Informatics at The Ohio State University since 2012, after receiving her Ph.D. from Princeton University. She is the recipient of the IEEE Signal Processing Society Young Author Best Paper Award in 2013 and the Best Paper Award at ICASSP 2012. She received the Young Investigator Program Awards from AFOSR and ONR respectively in 2015, the Ralph E. Powe Junior Faculty Enhancement Award from Oak Ridge Associated Universities in 2014, and Google Faculty Research Award in 2013. Her research interests include statistical signal processing, machine learning, information theory and their applications in high-dimensional data analysis, network inference, radar and bioinformatics.