
Dissertation Defense
Signal Detection with High-Dimensional Random Matrix Models
This event is free and open to the publicAdd to Google Calendar
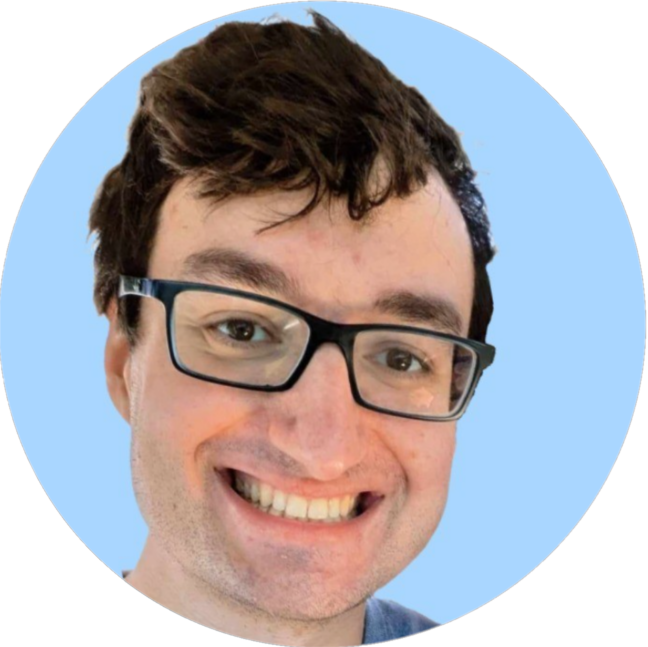
PASSCODE: Wigner
Advancements in computing over recent decades have enabled real-time processing of signals with hundreds to tens of thousands of thousands of features. However, in modern applications, the number of available samples often falls far short of the requirements of classical estimation and detection methods. In this talk, we explore techniques for high-dimensional signal detection through the lens of random matrix theory. Unlike classical large-sample asymptotics—where the number of features is fixed while the number of samples grows indefinitely—we focus on a high-dimensional asymptotic regime, where both the number of features and samples grow proportionally and approach infinity together. This setting exhibits the concentration of measure phenomenon, which gives rise to surprising universal behaviors in high dimensions that can be exploited for estimation and detection. After introducing key concepts from high-dimensional probability and random matrix theory (RMT), we overview some applications in statistical signal processing. In this talk, we show how RMT can be used to enhance signal detection in high dimensions, illustrating the theory for the applications of space-time adaptive processing (STAP), sequential change point detection, and community detection in temporal graphs. We demonstrate that, in the high-dimensional regime, biased estimators that exploit concentration of measure phenomena significantly outperform traditional estimators. These results highlight the practical advantages of high-dimensional techniques in modern signal processing and are supported by theoretical performance guarantees.
CHAIR: Professor Alfred Hero