
Dissertation Defense
Sequential Learning for Nanophotonics Inverse Design
Taigao Ma
WHERE:
1340 EECS (LNF Conference Room)Map
WHEN:
Wednesday, April 17, 2024 @ 1:00 pm - 3:00 pm
This event is free and open to the publicAdd to Google Calendar
This event is free and open to the publicAdd to Google Calendar
WEB: Event Website
SHARE:
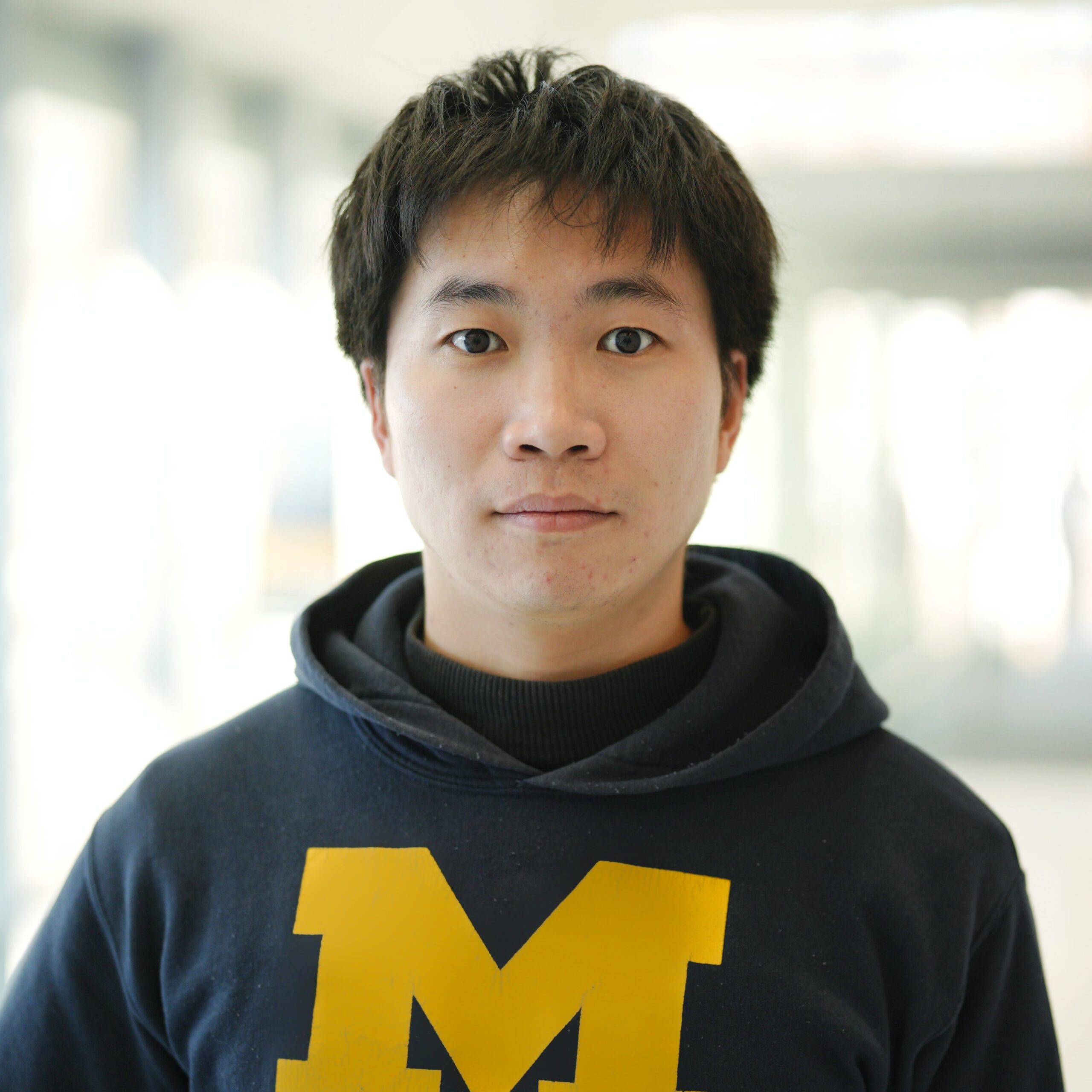
Nanophotonics plays an important role in enabling many practical applications. Inverse design deals with finding suitable nanostructures (a combination of material and structure dimensions) that can satisfy desired optical properties. Traditionally, optimization-based methods are widely used. However, they are not efficient as we need to restart the optimization process when facing new goals. Recently, many machine learning-based methods have been proposed to solve these problems. However, these models can only be designed for structure dimension, ignoring the material combinations.
This thesis explores two sequence-based methods: sequential decision process and conditional sequence generation, to tackle these inverse design challenges. First, I will talk about using traditional optimization to design multilayer structure and discusses about their drawbacks. Then, I will talk about how the inverse design can be treated as a sequence decision process and use the reinforcement learning algorithm OMLPPO to solve many practical photonic designs, including decorative chrome coatings, highly efficient filters for colorful solar cells, broadband transparent conductor, and ultra-broadband reflector. Finally, an efficient and universal algorithm based on conditional sequence generation will be proposed and discussed, including OptoGPT for inverse design and OL-Transformer for surrogate simulation.
CHAIR: Professor L. Jay Guo