
Faculty Candidate Seminar
Representation Learning for Health with Multi-modal Clinical Data
Add to Google Calendar
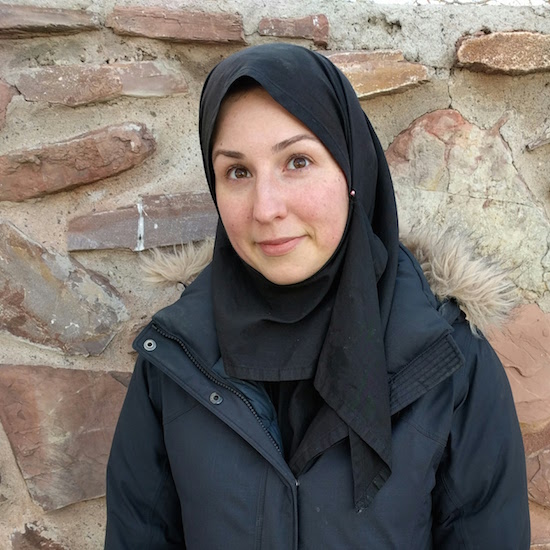
The explosion of clinical data provides an exciting new opportunity to use machine learning to discover new and impactful clinical information. Among the questions that can be addressed are establishing the value of treatments and interventions in heterogeneous patient populations, creating risk stratification for clinical endpoints, and investigating the benefit of specific practices or behaviors. However, there are many challenges to overcome. First, clinical data are noisy, sparse, and irregularly sampled. Second, many clinical endpoints (e.g., the time of disease onset) are ambiguous, resulting in ill-defined prediction targets.
I tackle these problems by learning abstractions that generalize across applications despite missing and noisy data. My work spans coded records from administrative staff, vital signs recorded by monitors, lab results from ordered tests, notes taken by clinical staff, and accelerometer signals from wearable monitors. The learned representations capture higher-level structure and dependencies between multi-modal time series data and multiple time-varying targets. I focus on learning techniques that transform diverse data modalities into a consistent intermediate that improves prediction in clinical investigation.
In this talk, I will present work that addresses the problem of learning good representations using clinical data. I will discuss the need for practical, evidence-based medicine, and the challenges of creating multi-modal representations for prediction targets varying both spatially and temporally. I will present work using electronic medical records for over 30,000 intensive care patients from the MIMIC-III dataset to predict both mortality and clinical interventions. To our knowledge, classification results on these task are better than those of previous work. Moreover, the learned representations hold intuitive meaning – as topics inferred from narrative notes, and as latent autoregressive states over vital signs. I will also present work from a non-clinical setting that uses non-invasive wearable data to detect harmful voice patterns, and the presence of pathological physiology.
Marzyeh Ghassemi is a PhD student in the Clinical Decision Making Group (MEDG) at MIT's Computer Science and Artificial Intelligence Lab (CSAIL) supervised by Dr. Peter Szolovits. Her research focuses on machine learning with clinical data to predict and stratify relevant human risks, encompassing unsupervised learning, supervised learning, structured prediction. Marzyeh's work has been applied to estimating the physiological state of patients during critical illnesses, modelling the need for a clinical intervention, and diagnosing phonotraumatic voice disorders from wearable sensor data.
While at MIT, Marzyeh was a joint Microsoft Research/Product intern at MSR-NE, and co-organized the NIPS 2016 Machine Learning for Healthcare (ML4HC) workshop. Her work has appeared in KDD, AAAI, IEEE TBME, MLHC, JAMIA, and AMIA-CRI. Prior to MIT, Marzyeh received B.S. degrees in computer science and electrical engineering as a Goldwater Scholar at New Mexico State University, worked at Intel Corporation, and received an MSc. degree in biomedical engineering from Oxford University as a Marshall Scholar.