
MIDAS Seminar
New methods for detecting natural selection in large samples of genetic data
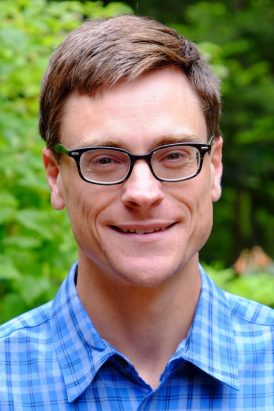
Understanding how humans evolved and adapted to their environment is one of the most important and interesting questions in science. The recent emergence of large, publicly available genetic data sets places the answers to these questions closer within reach than ever before. New statistical methods are needed to take full advantage of these resources.
In this talk Dr. Terhorst will discuss some recent progress towards detecting signals of recent natural selection in genetic data from tens of thousands of individuals. On the computational side, he will describe new memory- and compute-efficient inference algorithms that allow us to analyze thousands of genomes in parallel using GPUs. On the theoretical side, he will describe a new test for neutrality based on combinatorial properties of Kingman's coalescent. The test turns out to have interesting connections to a classic problem in theoretical statistics which has been studied by LeCam, Moran, Hall, and other luminaries. Some of this work is joint with Dan Erdmann-Pham, Kamm, Pier Palamara, Alkes Price and Yun Song.
Jonathan Terhorst joined the University of Michigan in the fall of 2017 as an assistant professor in the statistics department. Before that, he was a PhD student in statistics at UC Berkeley under the supervision of Prof. Yun Song. He is broadly interested in applications of statistics and machine learning to problems in biology, with a particular emphasis on statistical and population genetics.