
Communications and Signal Processing Seminar
l1 methods for tracking sparse signals
Add to Google Calendar
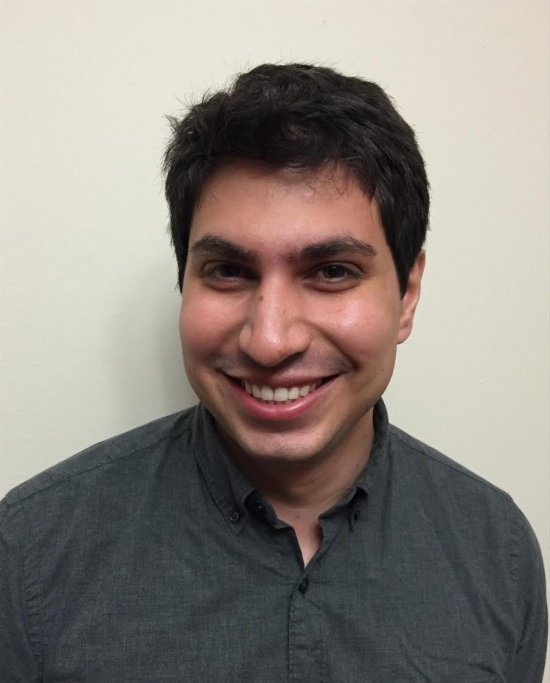
In the past two decades, the exploitation of low-dimensional models in signal processing has provided state-of-the-art performance in many important tasks. In particular, the most basic of such models – that the signal is sparse in a dictionary – has dramatically improved methods in image processing (e.g. de-noising, de-mixing) and efficient measurement acquisition (e.g. compressive sensing). Most applications that leverage sparsity, however, assume that the data is static. Often, however, our signals are intrinsically dynamic, and tracking these time-varying signals (i.e. target locations in a radar, or sequential frames in dynamic MRI) causally through time is necessary. This talk will thus focus on methods that aim to combine these two types of signal structure to provide accurate tracking performance. First, I will present simple algorithm, termed basis-pursuit de-noising with dynamic filtering (BPDN-DF), that combines optimization programs from both sparse- and dynamic-signal estimation and provide theoretical guarantees on the algorithm's performance over time. While effective in general settings, BPDN-DF lacks the robustness needed then to combat the statistics of dynamics-model-mismatch sometimes observed for sparse signals. Thus I will also present two additional algorithms, based on re-weighted l1 and the earth-movers distance (EMD), respectively, that aim to improve the robustness of tracking sparse signals under different noise conditions. The former of these methods, re-weighted l1 dynamic filtering (RWL1-DF) aims to improve the robustness of BPDN-DF to large, yet sparse, errors in the dynamics model. The latter, EMD dynamic-filtering (EMD-DF), aims to imbue a sparse coefficient space with a sense of geography, permitting dynamics models based on location to be used more effectively. One common thread in all these methods is a reliance on the dynamics function being known. For cases where the dynamics is unknown, I will demonstrate that a dictionary learning algorithm over the space of all dynamics functions can be used with BPDN-DF to learn a dictionary of dynamics for specific applications directly from data.
Adam S. Charles received both a B.E. and M.E in Electrical and Computer Engineering in 2009 from The Cooper Union in New York City, New York. He received his Ph.D. in Electrical and Computer Engineering from The Georgia Institute of Technology in 2015, where his research was awarded a Sigma Xi Best Doctoral Thesis award as well as an Electrical and Computer Engineering Research Excellence award. Currently, Adam is an NIH post-doctoral fellow at the Princeton Neuroscience Institute in Princeton New Jersey, where he studies theoretical and computational neuroscience. His research interests currently include neural imaging technologies, inference and tracking of sparse and structured signals, and statistical models of neural systems.