
Communications and Signal Processing Seminar
Flat Minima Generalize for Low-Rank Matrix Recovery
This event is free and open to the publicAdd to Google Calendar
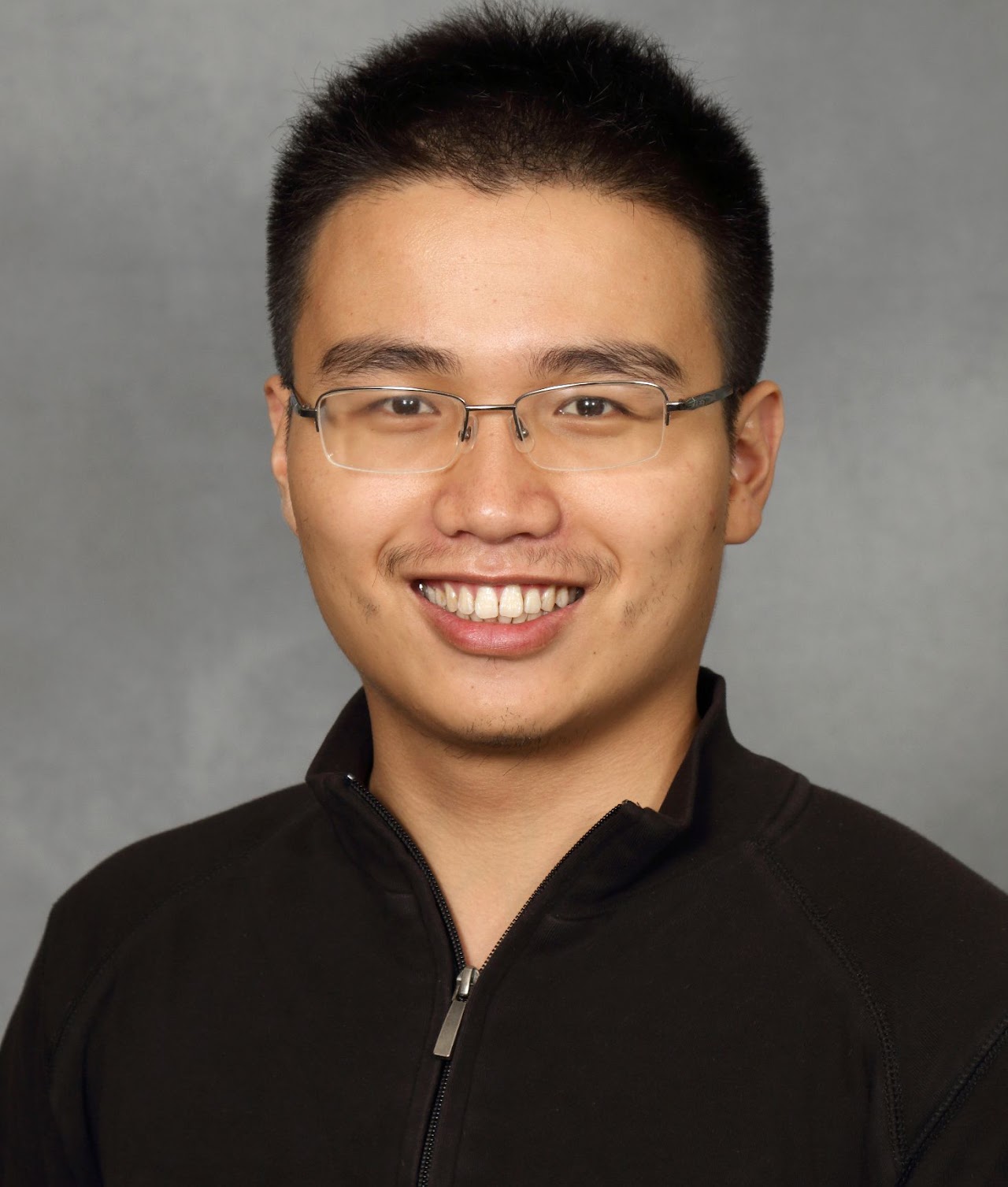
Abstract: Empirical evidence suggests that for a variety of overparameterized nonlinear models, most notably in neural network training, the growth of the loss around a minimizer strongly impacts its performance. Flat minima — those around which the loss grows slowly — appear to generalize well. This work takes a step towards understanding this phenomenon by focusing on the simplest class of overparameterized nonlinear models: those arising in low-rank matrix recovery. We analyze overparameterized matrix and bilinear sensing, robust PCA, covariance matrix estimation, and single hidden layer neural networks with quadratic activation functions. In all cases, we show that flat minima, measured by the trace of the Hessian, exactly recover the ground truth under standard statistical assumptions.
Bio: Lijun Ding is a post-doctoral scholar at the Institute for Foundations of Data Science (IFDS) at the University of Wisconsin and the University of Washington, supervised by Stephen J. Wright, Dmitry Drusvyatskiy, and Maryam Fazel. Before joining IFDS, he obtained his Ph.D. in Operations Research at Cornell University, advised by Yudong Chen and Madeleine Udell. He graduated with an M.S. in Statistics from the University of Chicago, advised by Lek-Heng Lim. He received a B.S. in Mathematics and Economics from the Hong Kong University of Science and Technology.
His research lies at the intersection of optimization, statistics, and machine learning. His work focuses on solving fundamental challenges and application problems in Data Science, where he develops optimization techniques that allow computational scalability and statistical methods that provide a better model of the structured data.
*** The event will take place in a hybrid format. The location for in-person attendance will be room 3427 EECS. Attendance will also be available via Zoom.
Zoom Passcode information is available upon request to Sher Nickrand ([email protected]).
This seminar will be recorded and posted to the CSP Seminar website.