
EECS Seminar
FFT-Accelerated and Tucker-Enhanced Parameter Extractors for Voxelized Structures
This event is free and open to the publicAdd to Google Calendar
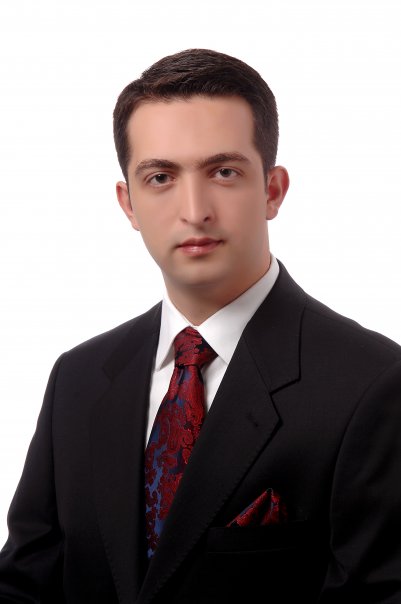
Abstract: Today, designers are extensively using parameter extractors during the designs of their chips, packages, integrated circuits, micro-electro-mechanical systems, and wireless charging units. While developing their designs via voxel-based virtual fabrication environments, which recently became popular to model unit process steps of the semiconductors and micro-electro-mechanical systems, designers are in need of parameter extractors that can be operated on interconnects/circuits/structures discretized by voxels (i.e., cubes). Furthermore, today’s 3D printing technology is voxel-based. To efficiently and accurately perform the analysis of 3-D printed boards, coils, and components, voxel-based analysis and design tools, particularly parameter extractors, are called for. In this seminar, I will talk about a series of integral equation-based parameter extractors for computing the capacitances, inductances, and impedances of interconnects and circuits discretized by voxels. These extractors were developed by exploiting the structured grid on which voxels reside and accelerated by fast Fourier transforms (FFTs) and specially developed preconditioners. Their memory and computational time requirements were drastically reduced by tensor decompositions, particularly Tucker decompositions. For voxelized structures, these extractors called VoxCap, VoxHenry/SuperVoxHenry, and VoxImp were proven to be much faster, more accurate, and more memory-efficient than their traditional fast and famous counterparts, FastCap, FastHenry, and FastImp, respectively. For the same level of accuracy in the analyses of voxelized structures, VoxCap, SuperVoxHenry, and VoxImp required 47x, 13x, and 55x less memory and 12x, 876x, and 16x less computational time compared to FastCap, FastHenry, and FastImp, respectively. On a desktop computer, VoxCap, SuperVoxHenry, and VoxImp successfully extracted the parameters of the structures requiring the solutions of linear system of equations with more than 100, 47, and 53 millions of unknowns, respectively. After introducing the Vox series, I will briefly talk about the DeepHenry, a deep learning-based extractor for inductance extraction of voxelized interconnects. I will share our results showing that deep learning-based DeepHenry is indeed 1157x faster than physics-based VoxHenry, while providing self/mutual inductances and self resistances of the interconnects with less than 4% error.
Bio: Abdulkadir C. Yucel is an Assistant Professor at Nanyang Technology University (NTU), Singapore, where he is currently developing physics-based and deep learning-based computational frameworks for the forward and inverse electromagnetic characterization. He received his M.S. and Ph.D. degrees in electrical engineering from the University of Michigan, Ann Arbor, MI, USA, in 2008 and 2013, respectively. He pursued his postdoctoral studies in various institutions, including the Massachusetts Institute of Technology, MA, USA. Dr. Yucel was the recipient of Fulbright Fellowship in 2006, EECS Departmental Fellowship of University of Michigan in 2007, and Honorable Mention Award at IEEE Int. Symp. AP-S in 2009. He is the author/co-author of 110 journal papers and conference papers/abstracts. He is a Senior Member of IEEE. He is currently serving as an Associate Editor for IEEE Antennas and Propagation Magazine and the International Journal of Numerical Modelling: Electronic Networks, Devices and Fields, as a reviewer for various technical journals, and as a reviewer and organizing committee member of several IEEE conferences.