
AI Seminar
Efficient Training and Inference in Deep Learning
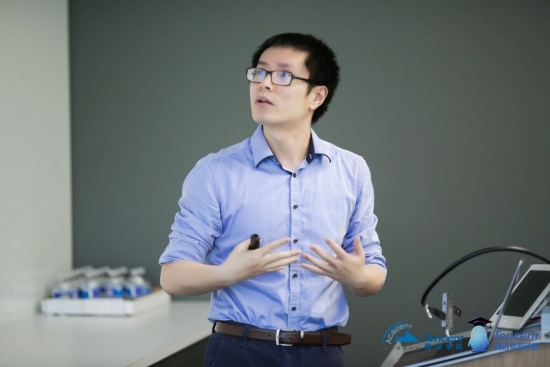
Training efficiency and inference efficiency are two crucial aspects to define the boundary of
deep learning in the practice. This presentation will introduce several recent parallel
optimization techniques done by Liu's group to improve the efficiency of distributed training in
deep learning, with a special focus on how to reduce the communication cost. The second part
of the presentation will cover several recent works on how to learn a hardware-aware deep
neural network for efficient inference.
Ji Liu is an assistant professor at University of Rochester and the director of Kwai AI lab at
Seattle. Ji Liu received his Ph.D. in computer science from University of Wisconsin-Madison. Before that he graduated from Arizona State University with the master degree in computer science and University of Science and Technology of China with the BS degree in automation, respectively. His research interests cover a broad scope of machine learning, optimization, reinforcement learning, and their applications in other areas such as data mining, healthcare, bioinformatics, computer vision, and other data involved areas. He won the award of Best Paper honorable mention at SIGKDD 2010, the award of Best Student Paper award at UAI 2015, and the IBM faculty award in 2017. He is also an awardee of MIT TR35 China 2017. He has published 60+ papers in top journals and conferences including JMLR, SIOPT, TPAMI, NIPS, ICML, TKDD, UAI, AISTATS, SIGKDD, ICCV, CVPR, etc.