
ECE Seminar
Distributed Learning, Control, and Inference in Large-Scale Systems.
This event is free and open to the publicAdd to Google Calendar
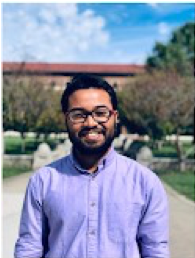
Abstract: Distributed autonomy and artificial intelligence have started shaping our lives like never before, be it in the form of the Internet-of-Things (IoT) or Google’s Federated Learning framework. As we strive to reap the benefits of these computing paradigms, their size and complexity pose various new challenges, dictating the need for advances in computation,communication, and control in the face of uncertain and unreliable components. Indeed, to envision smart infrastructure at a large scale, we must equip modern systems to adapt and react to model uncertainties, stringent communication constraints, andsophisticated adversarial attacks. In this talk, I will describe algorithms that address some of the above challenges for twobroad settings: (i) federated learning, and (ii) statistical inference over networks.
For the federated learning setting, I will develop a general algorithmic framework called FedLin that accounts for dataheterogeneity, slow and straggling devices, and compressed communication. Rigorous complexity guarantees will then bepresented for FedLin, showing that it converges linearly to the global minimum for smooth, strongly convex losses (as in acentralized setting), and that such linear convergence is preserved even under aggressive gradient sparsification. I will then derive a matching lower bound for FedLin, revealing that even mild statistical heterogeneity can hurt convergence rates.Notably, our work provides the first tight linear convergence rate analysis in federated learning.
In the second part of the talk, I will present a novel min-protocol for inference/hypothesis-testing over networks that departs fundamentally from existing “belief-averaging” schemes. I will then establish that the proposed min-rule guaranteesexponentially fast convergence to the true hypothesis (almost surely), with an asymptotic learning rate that strictly improvesupon all existing approaches. Furthermore, the proposed min-rule enjoys a simple extension that is provably robust to worst-caseByzantine adversarial attacks.
Overall, the algorithmic and theoretical insights from our work take a significant stride towards enabling reliable andefficient learning and decision-making in large-scale distributed systems.
Bio: Aritra Mitra is a Postdoctoral Researcher in the Department of Electrical and Systems Engineering, University of Pennsylvania. He received the Ph.D. degree from Purdue University, USA, the M.Tech. degree from the Indian Institute ofTechnology Kanpur, India, and the B.E. degree from Jadavpur University, Kolkata, India, in 2020, 2015, and 2013, respectively, all in Electrical Engineering. His current research interests include distributed learning and optimization, statistical signal processing, networked control systems, and security in multi-agent systems. He was a recipient of the University Gold Medal atJadavpur University and the Academic Excellence Award at IIT Kanpur.