
Communications and Signal Processing Seminar
Overparameterization and Global Optimality in Nonconvex Low-Rank Matrix Estimation and Optimization
This event is free and open to the publicAdd to Google Calendar
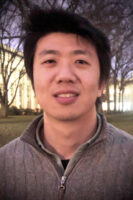
Abstract: Numerous important problems across communications and signal processing are reduced into nonconvex estimation/optimization over a low-rank matrix. In principle, these can be reliably solved to global optimality via convex relaxation, but the computational costs are prohibitive for use on real-world datasets. In practice, it is more common to optimize directly over the low-rank factors, in order to dramatically improve scalability, but at the cost of giving up on convexity. Unfortunately, large-scale algorithms like gradient descent often struggle to converge to a critical point and are always liable to fail completely by getting stuck at a spurious local minimum or saddle point. In this talk, I describe how rank overparameterization can overcome the nonconvexity of low-rank matrix estimation/optimization, in order to reliably and rapidly achieve global optimality. First,
overparameterization can be used to either certify global optimality or to generate a direction of escape. Second, given sufficient overparameterization, the nonconvexity becomes benign, in that every local minimum is a global minimum, and every saddle point can be escaped. Third, a simple preconditioner can greatly improve the convergence of gradient descent, particularly in the overparameterized regime. Finally, we examine large-scale computational results obtained on medical imaging datasets, recommendation engines, and neural network certification tasks.
Main related papers:
https://arxiv.org/abs/2207.01789
https://arxiv.org/abs/2206.03345 (joint work with Gavin Zhang and Salar Fattahi)
Bio: Richard Y. Zhang is an Assistant Professor in the Department of Electrical and Computer Engineering at the University of Illinois at Urbana-Champaign. He received the B.E. (Hons) degree with first-class honours in Electrical Engineering from the University of Canterbury, Christchurch, New Zealand, and the S.M. and Ph.D. degrees in Electrical Engineering and Computer Science from MIT. Before joining the Univerity of Illinois, he was a Postdoctoral Scholar at the University of California, Berkeley. His research interests are in optimization, machine learning, and applications in power and energy systems. He is particularly interested in theoretical foundations and practical algorithms for nonconvex low-rank matrix optimization and convex semidefinite programming. He is a recipient of the NSF CAREER Award in 2021.