
Communications and Signal Processing Seminar
Robust Learning by Double Over-Parameterization
This event is free and open to the publicAdd to Google Calendar
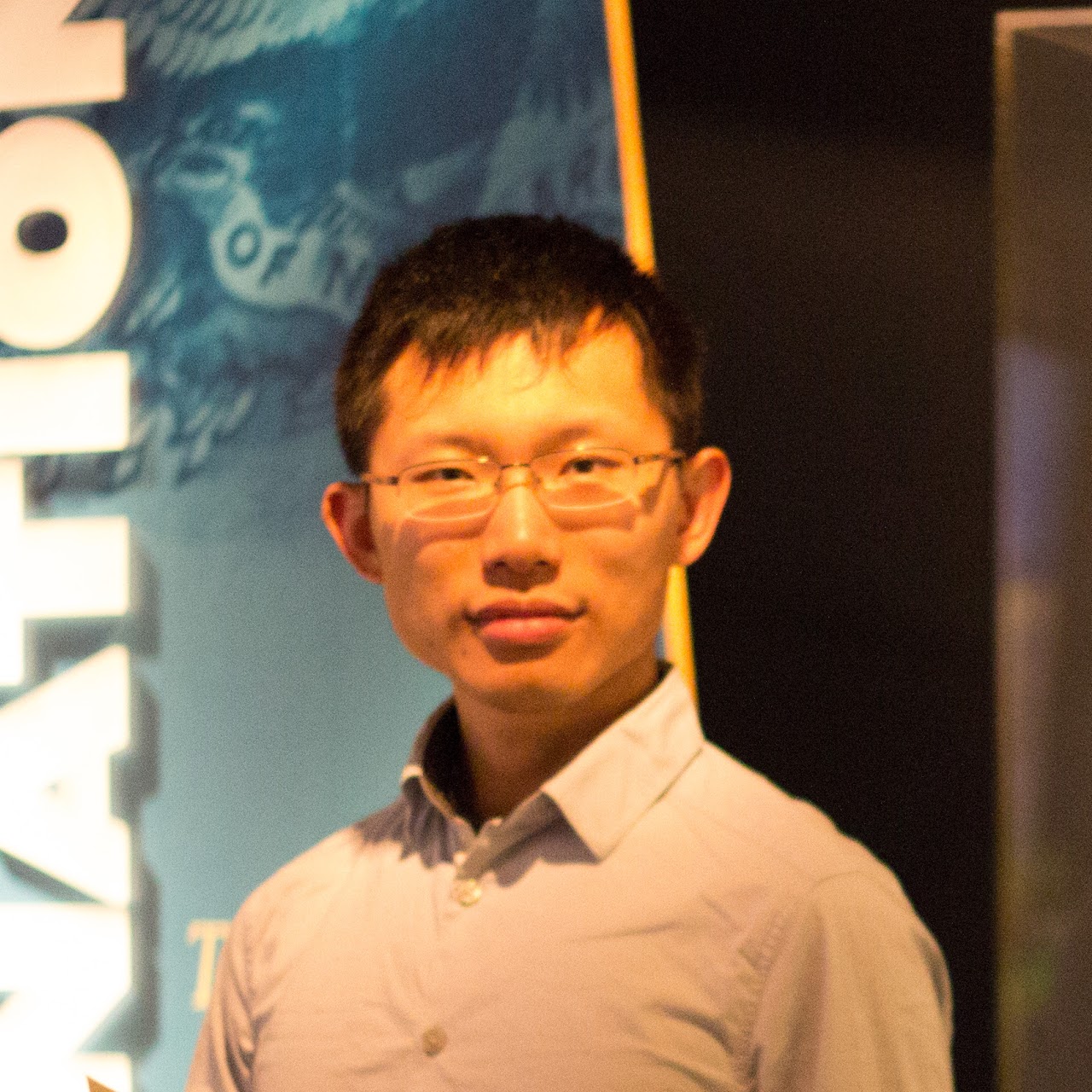
Abstract: Recently, over-parameterized models (e.g., deep neural networks) with more parameters than the amount of data have dominated the performances of modern machine learning. However, when the training data is corrupted, it is well-known that over-parameterized models overfit and do not generalize. While there exist many explicit regularizers such as weight decay for alleviating overfitting, they cannot fully separate data corruption and recover clean data.
In this talk I will show that overfitting to training data corruption can be addressed by introducing an extra over-parameterization term to the already over-parameterized model. Our method, called Double Over-Parameterization (DOP), contradicts classical wisdom that more parameters aggregate rather than alleviate overfitting. Crucially, overfitting is prevented by leveraging the implicit regularization of gradient descent with a particular choice of learning rates on different sets of model parameters. When applied to the tasks of image recovery from corrupted measurement and image classification under label noise, we show that DOP exhibits superior empirical performance. Finally, when applied to the task of robust principal component analysis, we prove that DOP can exactly recover a low-rank matrix from sparsely corrupted measurements, without prior knowledge on neither rank of the matrix nor sparsity of the corruption.
Bio: Chong You is a Research Scientist at Google NYC. Previously, he was a postdoc at UC Berkeley. He obtained his PhD in Electrical and Computer Engineering at Johns Hopkins University in 2018, and is the recipient of the MINDS Doctoral Dissertation Award. His research areas broadly include machine learning, computer vision, optimization and signal processing. He is particularly interested in sparse and low-dimensional modeling for the development of principled, efficient, and reliable algorithms (shallow & deep) for analyzing and interpreting modern data.
***Event will take place in hybrid format. The location for in-person attendance will be room 1008 EECS. Attendance will also be available via Zoom.
Join Zoom Meeting https: https://umich.zoom.us/j/91414297851
Meeting ID: 914 1429 7851
Passcode: XXXXXX (Will be sent via e-mail to attendees)
Zoom Passcode information is also available upon request to Michele Feldkamp ([email protected]).