
Dissertation Defense
Advances in Image Reconstruction for Digital Breast Tomosynthesis
Mingjie Gao
WHERE:
3316 EECS Building
WHEN:
Friday, December 8, 2023 @ 2:00 pm - 4:00 pm
This event is free and open to the publicAdd to Google Calendar
This event is free and open to the publicAdd to Google Calendar
WEB: Event Website
SHARE:
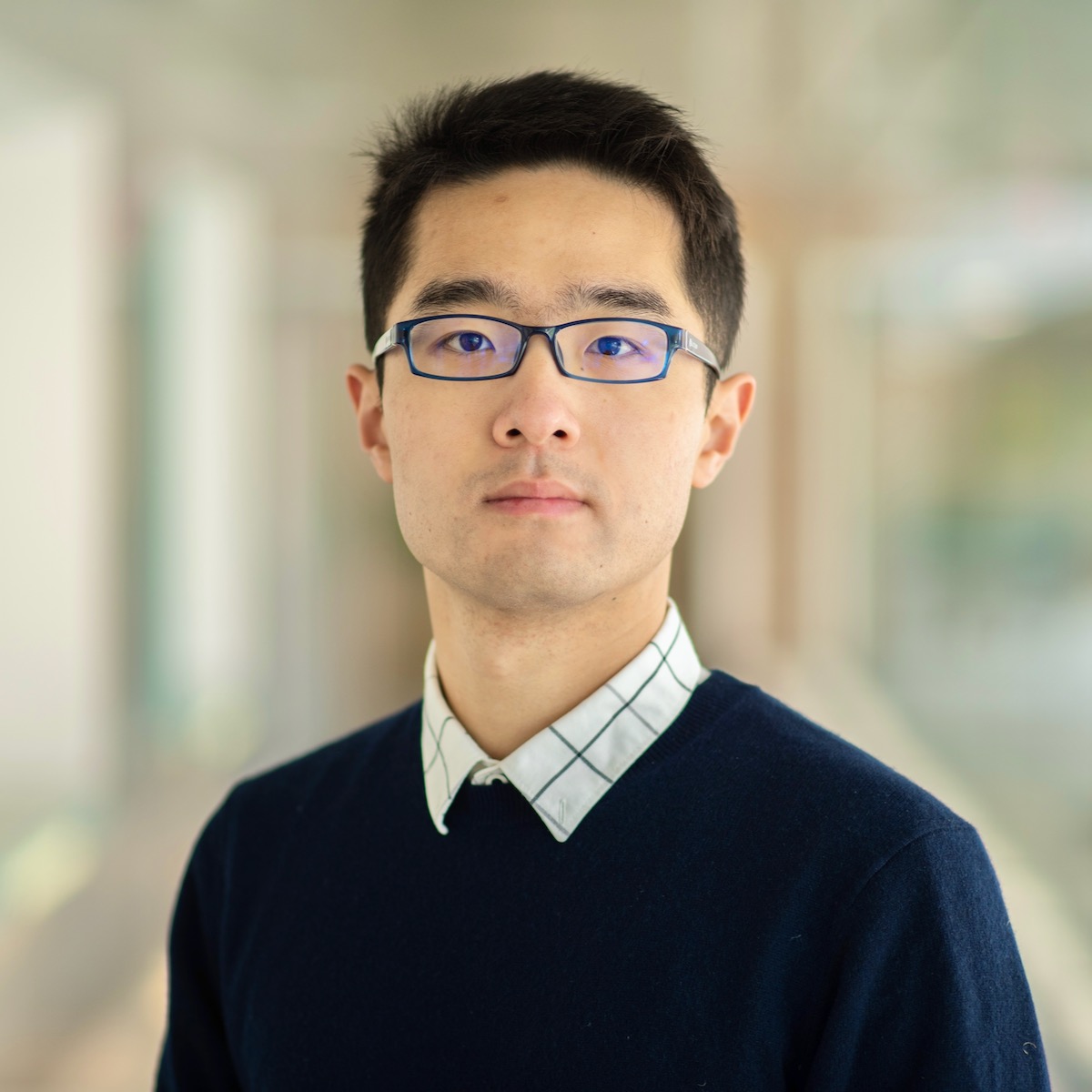
Passcode: 123456
Digital breast tomosynthesis (DBT) is a quasi-three-dimensional imaging modality that reduces the problem of overlapping tissues in two-dimensional mammography. It has significantly improved the diagnosis and treatment of breast cancer due to its high sensitivity and specificity in detecting microcalcifications (MCs), masses, and architectural distortions. However, DBT suffers from noise and blur problems that can compromise image quality and hinder the early detection of subtle cancers.
This dissertation is dedicated to enhancing the image quality and cancer diagnosis of DBT through image denoising, reconstruction, and deblurring. First, we developed a deep-learning-based image denoising method using convolutional neural networks (CNN) for post-reconstruction denoising. The denoiser improved the detectability index of MCs in human subject DBTs and the radiologists’ sensitivity in detecting phantom MC clusters in an observer study. Then, we integrated the CNN denoiser into model-based iterative reconstruction. Meanwhile, we designed a CNN model observer to assess the image quality based on the detection of MC clusters in human subject DBTs. The proposed reconstruction method increased the detection AUC of the CNN observer. Finally, we addressed the issue of x-ray source motion of DBT imaging system and developed a non-blind image deblurring method with a diffusion prior to improve the image resolution.
Co-Chairs: Professors Jeffrey A. Fessler and Heang-Ping Chan